One of the great problems in neuroscience is unraveling how the physical substrates of the brain develop, integrate, and organize to give rise to higher-order cognitive abilities as we experience the world. The burgeoning field of computational neuroscience uses mathematical models and computer simulations to tackle these tough questions, often with impressive results. This week, a French research team modeled the development of cognitive abilities using a three-level neurocomputational approach. Their model may reveal exciting insights into the biological and computational mechanisms of learning, and could have important applications to artificial intelligence moving forward.
🧠Article: “Multilevel development of cognitive abilities in an artificial neural network” (9/19/22) - Volzhenin, K., Changeux, J.-P., & Dumas, G. (2022). Multilevel development of cognitive abilities in an artificial neural network. Proceedings of the National Academy of Sciences of the United States of America, 119(38). https://doi.org/10.1101/2022.01.24.477526
🧠Introduction and Methods:
A powerful symbiotic relationship has blossomed between neuroscience and computer science as of late, with brain systems providing inspiration for prevalent computer algorithms like neural networks and computer-based mathematical models driving important research into the brain’s computational methods. Daniel Kahneman’s Thinking, Fast and Slow, has popularized the notion that human cognition is divided into distinct hierarchical systems, which Kahneman deems “system 1” and “system 2.” Artificial intelligence can handle system 1 tasks, pertaining to fast, nonconscious operations, just as efficiently as humans can. However, it still lags behind when it comes to system 2 tasks, which engage different cognitive pathways that are slower and enlist conscious deliberation.
The fact that computers can’t compete with humans at deliberate tasks means that computer scientists still have a lot to learn from the brain, which inspired researchers out of the Sorbonne to develop a computational model based on the most recent theories in human learning and cognitive development. They found that processes like synaptic pruning (the elimination of underused synapses), neurogenesis, and energy regulation, and accurate dopamine reinforcement were underrepresented in computational learning models. The team created a three-level computational framework that approximates the most up-to-date understanding of the brain’s learning processes, consisting of multiple hierarchical layers and simulating synaptic neuroplasticity and dopamine signaling. The lowest level of the model handles sensorimotor processing, which is analogous to raw perception. The second level handles global nonconscious processing, integrating information across different brain regions to handle “system 1” tasks. The final layer simulates a rudimentary degree of conscious processing, approximating that of the Drosophila fruit fly. This layer handles the more complex “system 2” tasks that computer programs tend to struggle with. Put together, the team hopes that this model will not only provide useful techniques for developing machine learning algorithms, but will also help shed light on how our brains develop complex cognition. Since this is a machine learning model, the algorithm self-updates to optimally perform the given tasks, which can produce results that surprise the researchers and hint at the hidden computational workings of the brain.
🧠Results and Conclusions:
The team ran their model through a series of behavioral tasks of increasing difficulty. As the tasks grew more complex, the researchers noted increasing levels of connectivity between the three levels of the system. The first task related to object recognition and was primarily handled by the sensorimotor layer, with minor assistance from the nonconscious layer of the model. To optimally perform the task, the model demonstrated a gradual pruning effect on almost two-thirds of the simulated synapses, while the remaining third was strengthened and stabilized. The learning process was nonlinear, with a rapid selection of synapses as the first ~200 images were being processed then a slower reinforcement period before plateauing at around a third of the initial synapse abundance at around ~1000 images (this is consistent with what we know about critical periods of postnatal learning in humans). The model selected for synapses based on how strongly each impacted the final output, with those that had the largest effect receiving the largest stability weighting.
To test the nonconscious cognition layer of the model, the team employed a delay conditioning task. In animal subjects, this kind of task is associated with the traditional classical conditioning process in which a unconditioned stimulus, like a loud noise, is associated with a conditioned stimulus, like a flashing light. Ultimately, the subject learns to produce the physiological response elicited by the loud noise in response to just the flashing light. In the computer model, images of numbers were used as both the conditioned and unconditioned stimuli. They showed that conscious processing is not necessary for the completion of this task, with connectivity between the simulated visual and motor cortices being sufficient to achieve over 95% accuracy with no recruitment of the conscious layer. Correct choices were rewarded by a simulated dopamine neuron, which the researchers equated to the striatum.
To test the conscious layer the team implemented a trace conditioning task, which mirrors classical conditioning except that there is a temporal delay between the presentation of stimuli in which the subject must hold a conscious image of the stimulus in mind. They found that simulating inhibitory neurons (which prevent other neurons from firing) was crucial for conscious processing, though many previous models have attempted to use only excitatory neurons. They also found that interneurons, which transfer signals between sensory and motor neurons, are crucial for creating and maintaining conscious mental representations of stimuli. This culminates in a functional model of working memory, a key element of conscious cognitive processing. Once again, simulated dopamine rewards were necessary to ascribe proper weighting to correct outcomes. Their model also autonomously generated spontaneous activity, or the ongoing activation of neurons that appear unrelated to the task being performed, which is associated with conscious processing in the human brain.
All three levels showed a reliance on synaptic pruning to achieve optimal results, emphasizing the importance of this biological mechanism in the learning process. The conscious level of the model performed optimally when it consisted of 20% inhibitory neurons, which has been predicted by previous theoretical work. Perhaps more interestingly, it reached optimal performance when generating 12 Hz of spontaneous activity, which overlaps closely with recorded measures of spontaneous activity in the human cerebral cortex. The goal of this study was to uncover biological mechanisms that optimize the learning process so that we can better understand the human brain and improve current models of artificial intelligence. To that end, the paper reveals the computational bases of dopamine reinforcement, inhibitory and interneuron function, synaptic pruning, and critical periods of learning to optimize cognitive performance.
Social touch has been shown to play a major role in maintaining physical health and mental well-being, but scientists have little to say about the underlying neural mechanisms of this crucial behavior. This week, a team out of Semmelweis University in Hungary discovered a neural pathway to the hypothalamus that motivates social contact and integrates with brain regions responsible for bonding, possibly revealing a clinical avenue for addressing disorders that affect social interaction.
🧠Article: “A thalamo-preoptic pathway promotes social grooming in rodents” (9/15/22) - Dávid Keller, Tamás Láng, Melinda Cservenák, Gina Puska, János Barna, Veronika Csillag, Imre Farkas, Dóra Zelena, Fanni Dóra, Stephanie Küppers, Lara Barteczko, Ted B. Usdin, Miklós Palkovits, Mazahir T. Hasan, Valery Grinevich, Arpád Dobolyi, A thalamo-preoptic pathway promotes social grooming in rodents, Current Biology, 2022, ISSN 0960-9822
🧠Introduction and Methods:
Sexual and nonsexual social touch are vital forms of communication in both rodents and humans. Previous work has shown that social sensory information follows a neural pathway from the thalamus to the cerebral cortex and that it is eventually processed in the hypothalamus (a primitive, pea-sized brain region that regulates instinctual and autonomic processes), but its specific route to the hypothalamus has always been a mystery.
Knowing this, Semmelweis researchers identified oddly-placed neurons that produce parathyroid hormone 2 in the thalamic nucleus. Parathyroid hormone 2, or PTH2, is a neuropeptide that has demonstrated a role in motivating mice to provide maternal care to their offspring. They also found that many of these neurons terminated in the medial preoptic area of the hypothalamus. Given this information, the team hypothesized that PTH2 was responsible for propagating sensory social signals from the thalamus to the hypothalamus, completing the missing link in the social touch pathway.
To test this idea, the team measured which neurons in the rat thalamic nucleus responded to social touch. They then stimulated these cells and measured the behavioral and neurobiological response using chemogenetics and anterograde viral tracing to visualize the neurons. Finally, they used autopsy material to verify the existence of a similar thalamic-hypothalamic pathway in human brains.
🧠Results and Conclusions:
The team found that mice that had been injected with excitatory receptors in the thalamic nucleus spent significantly more time performing social grooming. The excitatory treatment did not affect social behavior when the mice were separated by a glass divider, supporting the idea that thalamic nuclear neurons are specifically activated by physical social contact.
The team then used viral injections to tag the individual neurons that responded to social touch, and found that PTH2-producing neurons were selectively activated. The team found that when these tagged neurons were more active, the duration of social grooming behavior increased. To determine the pathway that underlies this behavior, the team used anterograde tracing to visualize the neuronal projections. They found that many thalamic PTH2 neurons terminate in the hypothalamus and have an excitatory effect on hypothalamic neurons in the medial preoptic area, which is consistent with our understanding of the role of the hypothalamus in processing social contact. Tracing methods in human brain tissue confirmed that we contain a similar pathway to the one identified here.
Ultimately, this study revealed the important role of the PTH2 neuropeptide in motivating social touch and identified a brand new neural pathway for this behavior. The team hopes that both of these discoveries might have clinical applications in treating mental disorders like autism, which come with deficits in social contact.
Brain-modeled neural networks called deep convolutional neural networks (DCNNs) represent the state of the art when it comes to artificially intelligent computer vision. Despite incorporating a three-dimensional structure based on the brain’s visual cortex, these popular models still fall woefully short of human visual perception and object recognition. Researchers out of York University sought to identify exactly why these programs underperform our brains, and to see if they could improve their results by modeling the algorithms even more closely after the human visual system.
🧠Article: Deep learning models fail to capture the configural nature of human shape perception (9/16/22) - Baker N, Elder JH. Deep learning models fail to capture the configural nature of human shape perception. iScience. 2022 Aug 11;25(9):104913. doi: 10.1016/j.isci.2022.104913. PMID: 36060067; PMCID: PMC9429800.
🧠Introduction and Methods:
One of the fascinating truths about neural network algorithms is that we simply don’t understand how they arrive at their conclusions. We can give them parameters and reinforce outcomes, but we don’t give them specific rules to solve a task. Because of this, the inner workings of these crucial systems often remain a mystery, leading computer scientists to label them a “black box.” This means that to refine an underperforming neural network, researchers have to devote major effort not just to solving the problem, but to identifying the problem in the first place. In the case of object recognition, a great way to do this is to examine how the program operates in comparison with the brain. Since the brain’s system of visual perception is still far superior to the best in computer vision, neuroscience is a crucial tool in developing the algorithms that will mold future technologies.
York University researchers sought to compare DCNNs to the visual cortex in relation to local and configural sensitivity, which is the ability to detect when certain parts of an image have been reversed in space or orientation. To test this, they compiled a set of animal silhouettes, some of which had been flipped in various ways. They then showed these data sets to humans and DCNNs, and attempted to improve DCNN performance by making the models more like the visual cortex.
🧠Results and Conclusions:
The study produced differing results when it came to local sensitivity versus configural sensitivity.
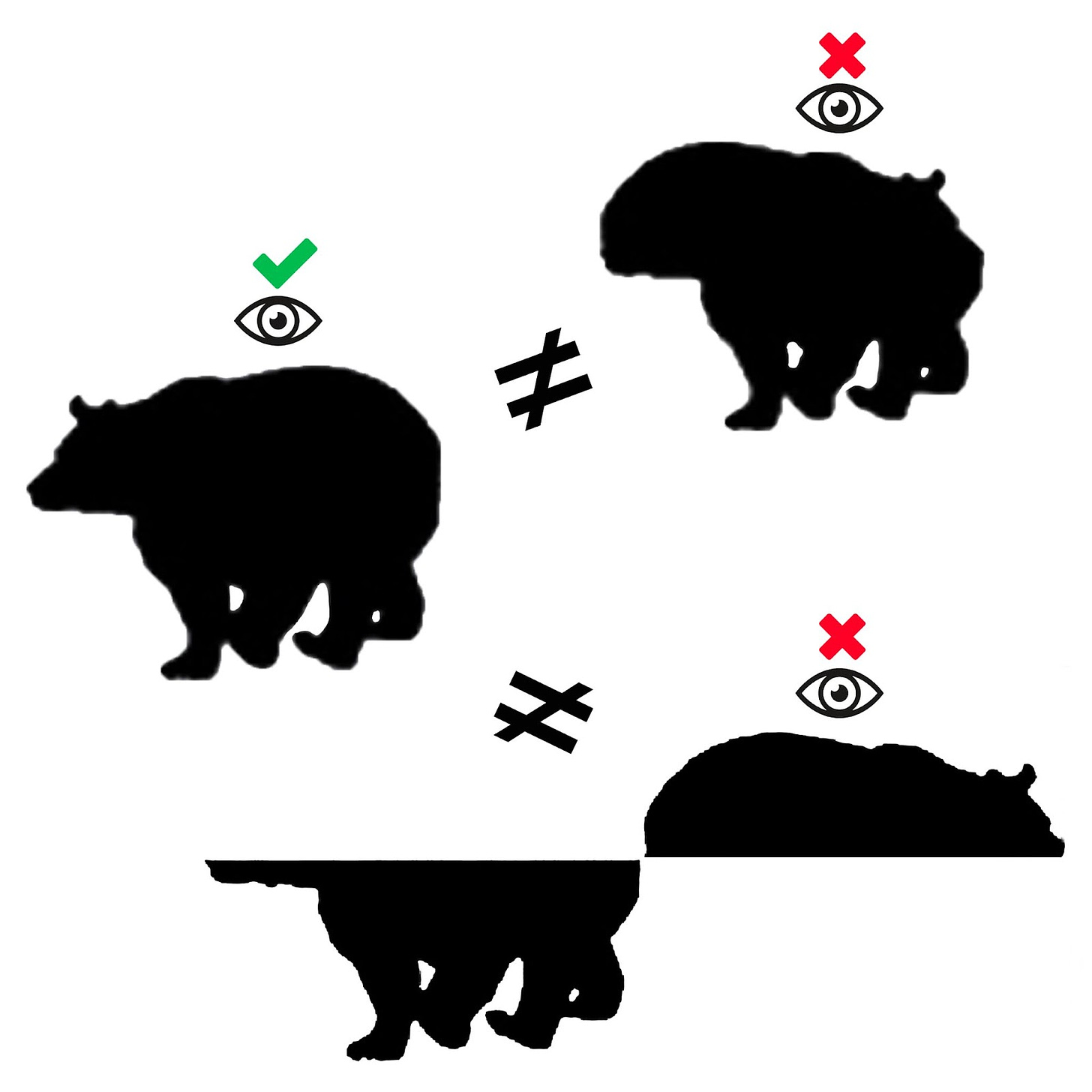
Objects are locally consistent when their location in space is the same, even if their orientation is altered. Objects are configurally consistent only when they have the same orientation. When it came to unaltered silhouettes, humans chose the correct classification 64% of the time while the algorithm performed at 47% accuracy. When the images were altered both locally and configurally, both the humans and the DCNN both performed far worse. However, when the images were changed configurally while remaining locally consistent, humans drastically underperformed their baseline while the algorithm’s accuracy was unaffected. If the models had shown an ability to discern configural changes to even a small degree, it would have meant that DCNNs may require only a series of incremental tweaks to match human visual processing. Instead, this complete insensitivity to configuration suggests that there is something fundamentally flawed about DCNNs as a classification model.
In spite of this, the researchers hoped that these algorithms could be improved by making them more similar to the human visual system. Research has shown that relative to humans, DCNNs weigh texture more highly than shape cues. The team tried to rectify this by choosing a model that was more sensitive to shape, but found no improvement in the DCNN’s ability to process configural changes. The researchers also tested a model which employed a series of feedback loops based on the primate visual cortex, but again saw no sensitivity to configuration.
Through this study, the team revealed a major discrepancy in visual processing when humans are compared to our most advanced and popular computer vision algorithms. While humans rely heavily on configural cues to classify visual stimuli, deep convolutional neural networks seem unable to make use of this information when recognizing an object. The researchers also showed that this problem does not have an obvious solution, meaning that there is a formidable new obstacle that must be overcome if DCNNs are to reach human-level visual processing anytime soon. Considering that these algorithms are used in everything from face recognition software to medical image analysis to object detection in self-driving cars, tackling configural sensitivity promises to be a pressing challenge for neuroscientists and computer scientists alike.
If you found value in our zero-cost information, you can support the newsletter by sharing it with a friend or by using the following links to purchase one of the curated neuroscience books we chose to review, Livewired by David Eagleman or The Body Keeps the Score by Bessel van der Kolk. Thanks for reading!